Challenges in Scaling AI Solutions Across Global Markets
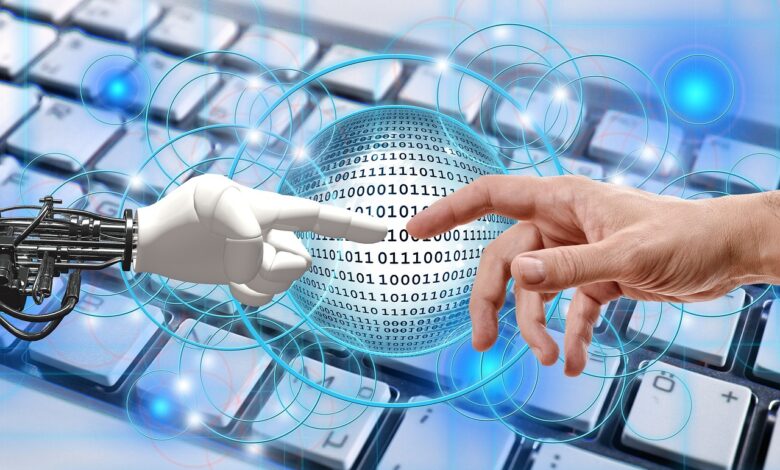
Artificial intelligence (AI) is changing the world, but scaling AI solutions globally is tough. Companies face many challenges when they try to expand AI worldwide. These include technical issues in new markets and adapting to different cultures and languages.
This article will look at the big hurdles companies face when they try to grow AI globally. We’ll cover technical, cultural, and legal challenges. Our goal is to help companies succeed in deploying AI worldwide.
Key Takeaways
- Navigating the technical infrastructure barriers in emerging markets is crucial for successful AI scaling.
- Cultural and linguistic adaptation requirements, such as language processing challenges and local user experience expectations, must be addressed.
- Ensuring regulatory compliance across different jurisdictions is a critical factor in global AI deployment.
- Data privacy and security considerations, including regional data protection laws and cross-border data transfer issues, must be carefully managed.
- Workforce skills and training requirements are essential for effective AI implementation in diverse global markets.
Understanding the Global AI Implementation Landscape
Artificial intelligence (AI) is becoming more popular around the world. It’s important to look at how AI is being used in different markets. By studying the global AI market, we can learn how to use AI technology well.
Current Market Distribution of AI Solutions
The AI market is changing fast. In North America, especially the United States, AI is used a lot. Europe and Asia-Pacific are also growing fast, thanks to new tech and understanding AI’s benefits.
Regional Technology Adoption Patterns
How quickly AI is adopted varies by region. In places like the United States, UK, and Japan, AI is used to innovate and improve business. In countries like India, China, and Brazil, AI is growing fast. They use it to solve problems and boost their economies.
Key Market Players and Stakeholders
The AI world has many players. Big tech companies like Amazon, Google, Microsoft, and IBM lead the market. They offer many AI solutions. Governments and researchers also play a big role, investing in AI and supporting its use.
Region | AI Market Share | Key AI Adopters |
---|---|---|
North America | 45% | United States, Canada |
Europe | 25% | United Kingdom, Germany, France |
Asia-Pacific | 20% | China, Japan, South Korea |
Rest of the World | 10% | Brazil, India, UAE |
Knowing how AI is used globally helps us see what’s next. It shows us the challenges and chances for AI to help us all.
Technical Infrastructure Barriers in Emerging Markets
As we look at AI solutions worldwide, we face a big challenge. Emerging markets struggle with poor internet, limited computing power, and old hardware. These issues make it hard to use advanced AI technologies smoothly.
One major problem is the lack of fast and reliable internet. In many places, the internet is slow and often goes down. This makes it hard to send data needed for AI to work well.
Another big issue is the lack of strong computing power. The hardware, like servers, can’t handle the complex AI tasks. This slows down AI systems and makes them less effective.
To solve these problems, we need new ways to improve the tech in these markets. We could invest in better internet, upgrade computers, and use edge computing. This helps process data faster and closer to where it’s needed.
By fixing these tech issues, we can make AI work better in more places. This way, AI can help people all over the world.
“Investing in robust technical infrastructure is crucial for unlocking the transformative power of AI in emerging markets.”
Cultural and Linguistic Adaptation Requirements
Expanding AI solutions globally means we must adapt culturally and linguistically. Understanding the unique tastes and needs of different markets is key. We face challenges like language processing and ensuring AI respects cultural norms. Meeting local user expectations is our top priority.
Language Processing Challenges
Deploying AI worldwide is tough due to language processing issues. It’s crucial to grasp and respond to language differences, idioms, and dialects. Advanced multilingual NLP is vital for AI to work well in diverse markets.
Cultural Nuances in AI Decision Making
AI must be aware of cultural sensitivities and norms. Decision-making factors differ greatly by region. AI needs to be trained to handle these differences responsibly. Local cultural knowledge is essential for AI to make decisions that match the audience’s values.
Local User Experience Expectations
- Tailoring the user interface and interaction design to match local aesthetics and interaction patterns
- Ensuring content and language is adapted to resonate with the target audience
- Integrating cultural references and metaphors that are meaningful and relevant to local users
- Addressing regional accessibility requirements and addressing potential barriers to adoption
By focusing on cultural and linguistic adaptation, we can fully realize AI’s global potential. This way, we offer personalized, intuitive experiences that connect with users everywhere.
Regulatory Compliance Across Different Jurisdictions
As companies grow their AI solutions worldwide, they face a big challenge. They must understand and follow many different rules. Knowing international AI laws well is key to avoiding problems.
One big issue is that AI rules vary a lot. Each country has its own way of handling AI, making it hard for companies to keep up. Keeping up with these changing AI regulations is a big job.
To grow AI globally, companies need to do a lot of research. They must know the latest laws and rules in each place they want to go. Working with legal experts can help avoid big problems and make sure they follow the rules everywhere.
Region | Key AI Regulations | Compliance Considerations |
---|---|---|
European Union | General Data Protection Regulation (GDPR), Proposed AI Act | Data privacy, algorithmic transparency, human oversight |
United States | Fragmented state-level regulations, proposed federal legislation | Bias mitigation, consumer protection, sector-specific guidelines |
China | Personal Information Protection Law (PIPL), Cyber Security Law | Data localization, cross-border data transfers, government oversight |
By tackling regulatory compliance head-on, companies can make the most of their AI efforts worldwide. This way, they can use these powerful technologies responsibly and ethically everywhere.
Data Privacy and Security Considerations
As AI solutions spread worldwide, keeping data safe is key. It’s important to follow local data laws and handle data transfers carefully. Also, setting strong security standards in different markets helps protect data and keeps users trusting.
Regional Data Protection Laws
Businesses must follow data laws like the GDPR in Europe and similar ones elsewhere. Knowing each area’s rules is vital to avoid fines and harm to reputation.
Cross-border Data Transfer Issues
Moving data across borders is a big challenge for AI users. Laws on data use and sharing can slow down AI systems. It’s important to plan well to meet these rules and keep AI working smoothly.
Security Implementation Standards
Protecting AI data and systems is crucial. Setting up the same security rules everywhere helps fight cyber threats. Working with local experts and using known security standards can help a lot.
Regulation | Key Requirements | Applicable Regions |
---|---|---|
GDPR |
|
European Union |
CCPA |
|
California, United States |
APEC CPRS |
|
Asia-Pacific Economic Cooperation (APEC) region |
By focusing on data privacy and security, companies can earn trust and reduce risks. A good plan that covers local laws, data transfers, and strong security is key. This helps navigate the complex world of data protection with AI.
Resource Allocation and Cost Management
Scaling AI solutions globally means you need to plan your resources and manage costs well. Deploying AI in many markets requires a smart budget and resource plan. As you grow your AI globally, you’ll face challenges with AI implementation costs, resource planning, and global expansion budgets.
One big challenge is figuring out the costs of AI deployment. Costs include setting up infrastructure, managing data, hiring talent, and keeping it all running. Budgeting and forecasting are key to keeping your AI rollout affordable and sustainable.
Also, each market has its own needs for resources. Things like local talent, infrastructure, and rules can affect your planning. Being able to adjust your resource plan for each region can help you make the most of your AI investment.
Cost Category | Estimated Range |
---|---|
AI Platform and Infrastructure | $50,000 – $500,000 |
Data Collection and Preprocessing | $10,000 – $100,000 |
Model Training and Deployment | $20,000 – $200,000 |
Ongoing Maintenance and Support | $5,000 – $50,000 per year |
By focusing on resource planning and AI implementation costs, you can manage your global expansion budget better. With smart resource allocation, cost cutting, and flexible budgeting, you can make AI work for you worldwide.
Integration with Legacy Systems Worldwide
Businesses worldwide are trying to use artificial intelligence (AI) to boost innovation and efficiency. But, they face a big challenge: integrating AI with their old systems. It’s key to make AI and legacy systems work together smoothly to unlock their full power.
System Compatibility Issues
One big problem is making AI systems work with the many old software and hardware that companies use. Old protocols, special interfaces, and different data formats can block AI integration. To solve this, you need to understand both AI and old systems well and plan how to update them.
Migration Strategies and Challenges
Switching from old systems to AI-friendly ones is another big challenge. This process includes changing data, rewriting apps, and updating infrastructure, all while keeping business running. Good migration plans must consider legacy system integration, AI compatibility, and system migration to make the transition smooth and beneficial.
Compatibility Challenges | Migration Strategies |
---|---|
|
|
By tackling these integration and migration hurdles, companies can fully use AI globally. This leads to better efficiency, innovation, and a strong competitive edge.
Workforce Skills and Training Requirements
As companies try to grow their AI globally, they hit a big roadblock – the AI skills gap. There aren’t enough AI experts, especially in new markets where tech is getting more advanced. To solve this, companies must focus on finding and training the right talent worldwide.
Finding the right people is key for AI success. Companies need to look globally for talent in areas like machine learning and data engineering. This way, they can fill the AI skills gap and have a skilled team to lead AI projects.
Training the workforce is also vital. Offering detailed training to local teams is crucial for a strong AI system. These programs should teach AI tech, ethics, and cultural awareness for global markets.
Workforce Training Strategies | Key Benefits |
---|---|
|
|
By tackling the AI skills gap with global talent acquisition and workforce training, companies can build the skills needed to grow AI worldwide.
“The key to unlocking the full potential of AI lies in developing a skilled and adaptable workforce that can navigate the unique challenges of global AI deployment.”
Market-Specific AI Model Adaptation
In today’s fast-changing AI world, making these technologies work well in different markets is a big challenge. We need to adapt AI models for each market’s unique data, culture, and user needs. This ensures they perform well and meet user expectations.
Local Data Collection Methods
To start adapting AI models, we must collect the right data from each market. This data helps us train and improve our AI models. We can use various methods, such as:
- Crowdsourcing data from local users
- Working with local partners for exclusive data
- Using machine learning to scrape and annotate data
These data collection strategies help our AI models understand and serve each local market better.
Model Retraining Protocols
After collecting local data, we need to retrain our AI models. This model retraining process can include:
- Transfer learning, where we adjust pre-trained models with local data
- Incremental learning, for ongoing updates and improvements
- Adversarial training, to make models more resilient to cultural or linguistic differences
By using theseAI model localizationmethods, we can make sure our AI solutions work well in many different markets.
Localization Technique | Description | Key Benefits |
---|---|---|
Transfer Learning | Fine-tuning a pre-trained model using local data | Faster model development, improved accuracy |
Incremental Learning | Continuously updating the model as new data becomes available | Maintains relevance and performance over time |
Adversarial Training | Training the model to be robust against cultural or linguistic variations | Enhances model generalization and adaptability |
“Effective AI model localization is the key to unlocking the true potential of these transformative technologies in diverse global markets.”
Challenges in Scaling AI Solutions Across Global Markets
Organizations face many challenges when they try to use AI technology worldwide. They need to adapt to different cultures and rules. They also have to make sure AI works well with old systems. This is a tough journey that needs careful planning and action.
One big problem is that technology adoption varies by region. Some places quickly take up new AI tech, while others struggle with the basics. To succeed, you need a plan that fits each market’s unique needs.
Another challenge is making AI solutions fit local tastes and ways of communicating. If AI doesn’t match local preferences, it won’t work well. This can make users unhappy and reduce AI’s effectiveness.
- Adapting AI models to local data collection methods and retraining protocols
- Ensuring compliance with diverse regulatory frameworks and data privacy laws
- Aligning AI-powered solutions with culturally specific ethical frameworks
To overcome these hurdles, a team effort is needed. It should include experts from different fields, partners, and local people. With a well-rounded plan, companies can tackle the challenges of AI on a global scale.
“Scaling AI solutions across global markets is not a one-size-fits-all endeavor. It requires a deep understanding of local nuances, a commitment to adaptability, and a willingness to collaborate with stakeholders from diverse backgrounds.”
Performance Monitoring and Quality Assurance
AI solutions are growing worldwide, making it key to keep performance and quality high everywhere. Our company has a detailed plan to tackle this challenge. We use top-notch monitoring systems and strong quality checks to make sure AI works well everywhere.
Our AI performance metrics dashboard gives us live updates on how our AI is doing globally. We watch things like how accurate it is, how fast it responds, and how users interact with it. This helps us spot and fix any issues fast, making sure our AI meets top standards everywhere.
Our team also does regular global monitoring of AI use. They look at lots of data to find problems or areas to get better. This way, we can keep our AI up to date and tailored to local needs.
By focusing on keeping AI performance and quality high, we meet our clients’ high expectations, no matter where they are. This focus on quality sets us apart in the global AI market.
“Effective performance monitoring and quality assurance are essential for scaling AI solutions across global markets. It’s the foundation that allows us to maintain consistent excellence, no matter the location.”
Ethical Considerations in Global AI Deployment
Scaling AI solutions worldwide brings up big ethical questions. We must make sure AI systems fit with different cultural values. It’s also key to use AI in a way that’s fair and respectful of all cultures.
Cultural Ethics Alignment
AI solutions need to understand local cultural and ethical norms. What’s right in one place might not be in another. We must study these differences and make our AI systems reflect the values of the communities they help.
Bias Prevention Strategies
Bias is a big problem in AI, especially when we use it globally. We need strong plans to stop bias, taking into account different cultures and languages. This includes using diverse data, checking AI algorithms, and watching for new biases.
Bias Prevention Strategies | Description |
---|---|
Diverse Data Collection | Make sure the data for AI models shows the diversity of the people it will help. This includes different cultures, demographics, and languages. |
Algorithmic Auditing | Use strict checks to find and fix biases in AI algorithms before we use them. |
Continuous Monitoring | Keep an eye on AI systems for new biases. Change them as needed to keep AI fair and responsible. |
By focusing on AI ethics, cultural sensitivity, and bias prevention, we can make sure our global AI efforts are not just about tech. They also respect the well-being of diverse communities everywhere.
Partnership and Vendor Management
As companies grow their AI solutions worldwide, managing partnerships and vendors is key. Building strong global partnerships and handling various vendor relationships is a big challenge. It needs a smart plan to keep quality high and foster international collaboration.
Finding the right partners and vendors is essential. They should share your vision and meet local market needs. Look at their technical skills, cultural fit, and ability to grow. Good communication and clear roles are vital for teamwork and success.
Setting up strong quality checks is also important. This might include standard tests, audits, and tracking performance in different places. Fixing problems quickly and always looking to improve helps keep services high and customers happy everywhere.
Key Considerations in Global Partnership and Vendor Management |
---|
|
By tackling these challenges with a smart plan, companies can make their AI solutions work well worldwide. This means they can grow and succeed in many markets.
“Effective partnership and vendor management is crucial for scaling AI solutions globally. It requires a strategic, adaptable approach to ensure consistent quality and alignment across diverse markets.”
Future-Proofing Global AI Solutions
As AI technology keeps changing fast, we must make our global AI solutions ready for the future. This means planning for growth and keeping up with new tech. By being proactive, we can keep our AI offerings useful and effective worldwide.
Scalability Planning
Scalability is key for AI solutions to succeed over time. We need to design our systems to handle more data, users, and growth globally. This includes using distributed computing, cloud infrastructure, and modular design. By focusing on scalability, we can make our AI solutions ready for future challenges and chances.
Technology Evolution Adaptation
The AI world is always changing, with new discoveries and tech coming fast. To keep our AI solutions future-proof, we must stay flexible and up-to-date. We should watch industry trends, learn about new tech, and add it to our products. By always improving and innovating, we can keep our AI solutions moving with the tech world.
Key Considerations for Future-Proofing AI Solutions | Strategies for Success |
---|---|
|
|
By focusing on AI scalability, technology adaptation, and long-term planning, we can make our global AI solutions ready for the future. This ensures their success in a world that’s always changing.
“The future belongs to those who can embrace change and adapt to the evolving technological landscape. By future-proofing our AI solutions, we can stay ahead of the curve and deliver unparalleled value to our global customers.”
We’ve looked into the challenges of scaling AI solutions worldwide. This journey is complex, needing a smart and flexible strategy. It involves overcoming technical hurdles in new markets and understanding local cultures and languages.
Looking ahead, companies wanting to grow their AI globally must focus on following local laws, protecting data, and keeping it safe. They also need to update old systems and train workers to use AI in new ways.
By tackling the challenges of AI globally, companies can set themselves up for success. The journey won’t be easy, but with the right approach, AI’s future looks bright. It promises innovation and growth in many markets.
FAQ
What are the current market distribution and regional technology adoption patterns for AI solutions globally?
We look at how AI is used worldwide. We see how different places adopt AI at different rates. We also find out who the big players are in the global AI world.
What are the key technical infrastructure barriers in emerging markets that can hinder AI implementation?
We talk about the tech problems in new markets. These include bad internet, old computers, and limited power. We share ways to solve these issues to help AI grow.
How do companies need to adapt AI solutions to accommodate cultural and linguistic differences across global markets?
We dive into making AI work for different cultures and languages. This includes dealing with language barriers and making sure AI is respectful of local customs.
What are the regulatory compliance requirements and legal frameworks companies need to navigate when scaling AI solutions globally?
We look at the laws for AI in different countries. We talk about the rules, the challenges, and how to follow them when AI goes global.
What are the critical data privacy and security considerations when deploying AI solutions worldwide?
We discuss keeping data safe and private when AI goes global. This includes local laws, moving data, and keeping it secure everywhere.
How can companies effectively manage the resource allocation and cost challenges of scaling AI solutions globally?
We tackle the money and resource issues of growing AI worldwide. We share ways to manage costs and plan for AI growth globally.
What are the key integration challenges when incorporating AI solutions with existing legacy systems in different global markets?
We talk about the hard part of adding AI to old systems worldwide. This includes making sure they work together and finding ways to integrate smoothly.
What are the workforce skills and training requirements for successfully scaling AI solutions globally?
We focus on the people side of growing AI worldwide. We discuss finding skilled workers, hiring, and training local teams.
How can companies adapt AI models to perform effectively in specific global markets?
We explain why AI needs to be tailored for each market. This includes using local data, retraining models, and making sure AI works well everywhere.
What are the key challenges in maintaining consistent AI performance and quality assurance across global markets?
We explore keeping AI the same everywhere. We share ways to monitor, ensure quality, and improve AI globally.
What are the ethical considerations and cultural alignment requirements when deploying AI solutions globally?
We address the ethics of AI worldwide. We talk about respecting local values, avoiding bias, and being responsible with AI globally.
How can companies effectively manage partnerships and vendor relationships when scaling AI solutions internationally?
We discuss the challenges of working with partners and vendors globally. We share strategies for good international partnerships, managing suppliers, and keeping quality high.
How can companies future-proof their global AI solutions to ensure long-term success and adaptability?
We talk about making AI solutions last in the global market. We discuss planning for growth, keeping up with tech changes, and staying relevant worldwide.